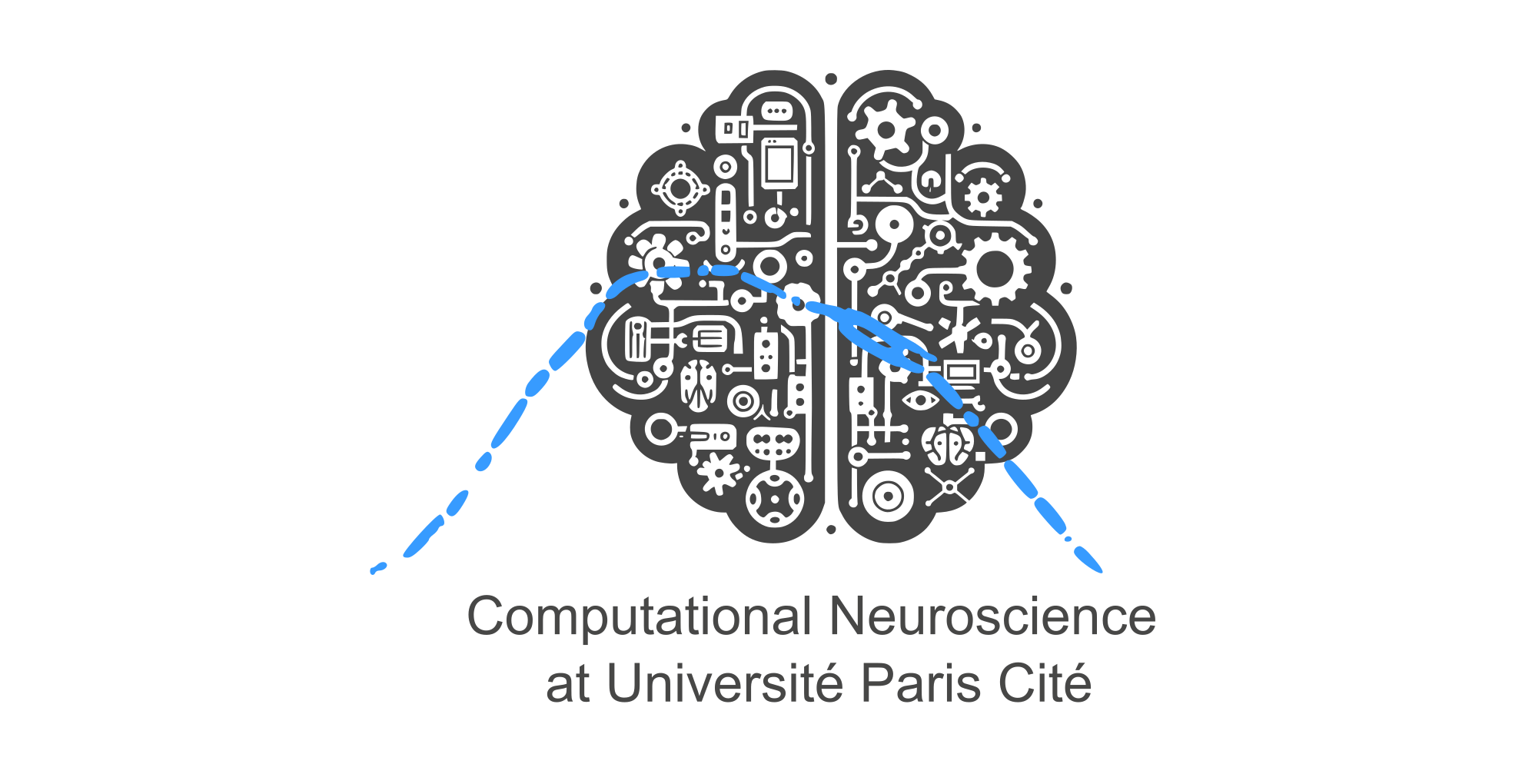
© Tous droits réservés
Program
9h – Introduction
9h15 – Samuel Bottani, Professor in Physics
Lab: Integrative Neuroscience and Cognition Center (INCC, CNRS) – Science Faculty
Title: Artificial Neural Networks: from cultures to in silico
9h45 – Laura Dugué, Professor in Cognitive and Computational Neuroscience
Lab: Integrative Neuroscience and Cognition Center (INCC, CNRS) – Science Faculty
Title: Models of cortical traveling waves
10h15 – Coffee Break
10h30 – Michael Graupner, CNRS Researcher in Experimental and Computational Neuroscience
Lab: Saints-Pères Paris Institute for the Neurosciences (SPPIN, CNRS)
Title: Natural firing patterns reduce sensitivity of synaptic plasticity to spike timing
11h – Iris Menu, Associate Professor in Cognitive Psychology
Lab: Institut de Psychologie (LaPsyDÉ, CNRS) – SHS Faculty
Title: Preterm birth and neurocognitive developmental trajectories
11h30 – Florent Meyniel, CEA Research Director in Cognitive and Computational Neuroscience
Lab: Unité de neuroimagerie cognitive – Computational Brain Team (INSERM/CEA/GHU)
Title: A computation approach to learning and decision making in the brain
12h – Lunch break
13h30 – KEYNOTE – Heike Stein, CNRS Researcher in Computational Neuroscience
Lab: Institut des Systèmes Intelligents et de Robotique (ISIR, CNRS & Sorbonne Université)
Title: Data-driven modeling of neural and behavioral dynamics
14h30 – Coffee Break
14h45 – Alessio Quaresima, Post-doc in Computational Neuroscience
Lab: Institut de l’Audition, Institut Pasteur – Bathellier Lab
Title: Inhibitory plasticity modulates adapting and persistent responses in cortical networks
15h15 – Jonathan Vacher, Associate Professor in applied Maths
Lab: MAP5 (CNRS) – Science Faculty
Title: Generative models to guide vision studies
15h45 – Coffee Break
16h – Round table
How do we federate, and for what purpose, the local community in computational neuroscience? And how do we coordinate with other local and national initiatives?
Keynote Guest Speaker
Heike Stein
CNRS Researcher in Computational Neuroscience, ISIR CNRS and Sorbonne Université
Abstract
Traditionally, computational neuroscience has been focused on proposing “hand-tuned,” biologically constrained models of neural dynamics. More recently, data-driven modeling approaches have gained in popularity and led to new theoretical insights, enabled by unprecedented access to large-scale neural and behavioral data, as well as fast-paced progress in machine learning and AI.
In the first part of this talk, I will lay out how dimensionality reduction methods can shine light on the nature of neural population dynamics by capturing covariance in large datasets. Commonly, such methods search for patterns of coactivation across neurons, which can describe correlated neural variability in fixed, low-dimensional subspaces. In contrast, I will present a novel method called slice tensor component analysis (sliceTCA) that can identify higher-dimensional structure in neural data, including stereotyped neural sequences or slowly evolving latent spaces during learning.
In the second part, I will present behavioral models of data from a novel locomotor task where mice learn coordinated gaits on a complex surface. To study the dynamical principles of locomotor learning, we modeled gait dynamics by fitting coupled-oscillator models to the behavioral time series. Switching dynamical regimes allowed us to extract lawful dynamics despite frequent adjustments of stepping patterns to the complex surface. With this approach, we find that coordinated locomotion on complex surfaces depends on a dual learning process: On the one hand, mice learned to use advantageous, naturalistic stepping patterns more extensively; on the other hand, dynamic entrainment between paws increased, leading to efficient, fine-grained coordination between paws. Together, this novel approach demonstrates how explicit modeling of behavioral time series can provide mechanistic insights into the dynamics of animal behavior and learning.